In the fast-paced world of modern data management, Vector Databases and Vector Search have emerged as transformative technologies, revolutionizing the way we store and retrieve information. This article provides an in-depth exploration of what Vector Databases and Vector Search are, how they work, and delves into practical use cases and examples that highlight their real-world applications.
Table of Contents
ToggleWhat is a Vector Database?
A Vector Database is a specialized type of database designed to handle high-dimensional data by utilizing vectorization techniques. Unlike traditional relational databases that rely on tabular structures, Vector Databases represent data points as vectors in a multi-dimensional space. This approach allows for more efficient storage, retrieval, and analysis of complex, unstructured data.
How it Works: Vectorization And High-Dimensional Spaces
Vectorization involves converting data points, such as text, images, or numerical values, into vectors. These vectors are then organized in a high-dimensional space, where the relationships between data points are preserved. The database leverages mathematical operations to navigate and analyze this space, enabling quick and accurate queries even in large datasets.
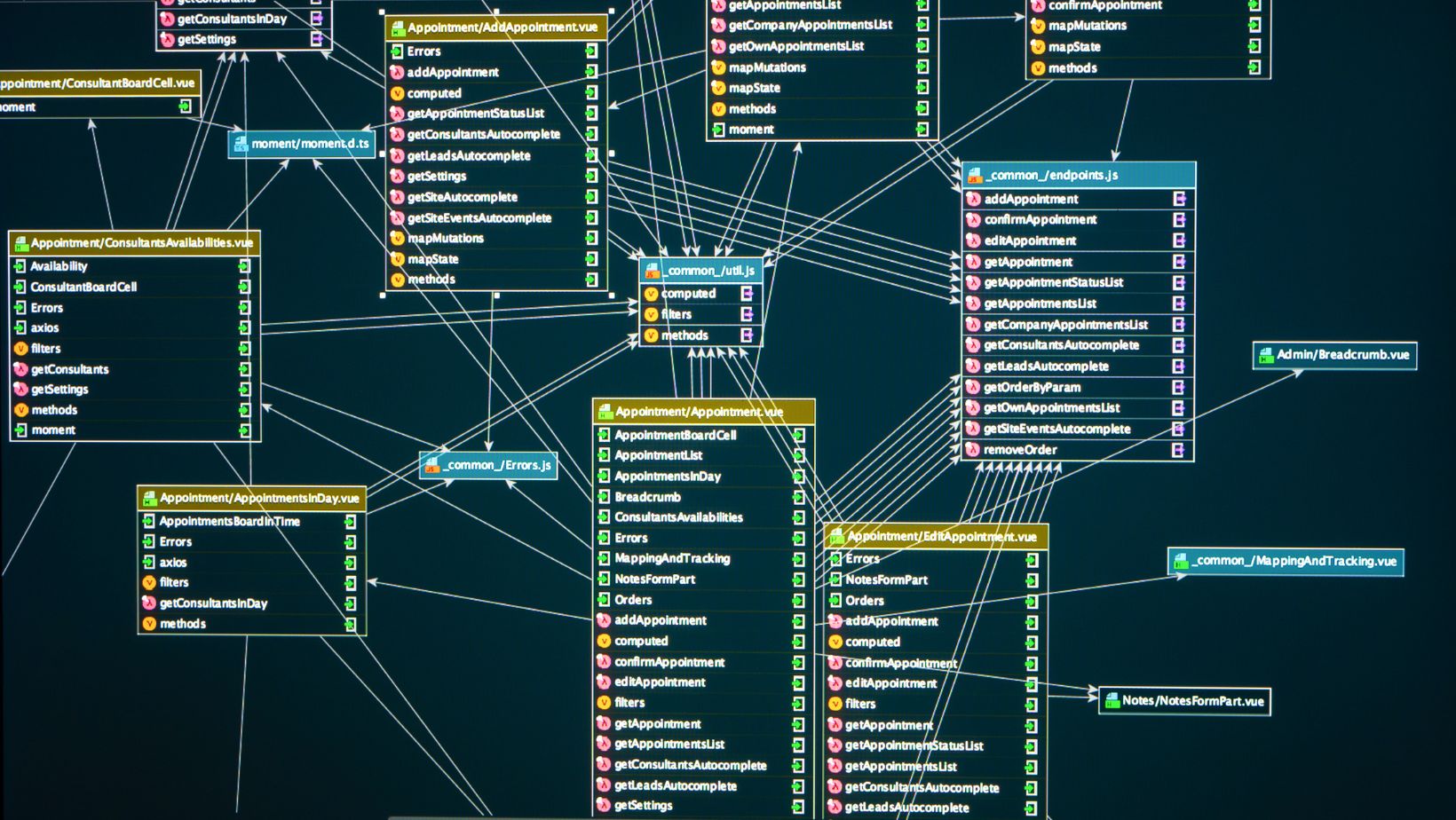
What is Vector Search?
Vector Search, also known as Similarity Search, is an advanced search technique that goes beyond traditional keyword-based searches. Instead of relying on exact matches, Vector Search evaluates the similarity between vectors, allowing for more context-aware and nuanced search results. This technology is particularly effective in applications where understanding the relationships between data points is crucial.
How it Works: Semantic Understanding And Real-Time Insights
Vector Search works by measuring the distance or similarity between vectors in the high-dimensional space. This approach allows for a more semantic understanding of data, enabling the system to provide relevant results based on the context of the query. The real-time nature of Vector Search ensures swift responses, making it ideal for applications that demand instantaneous insights.
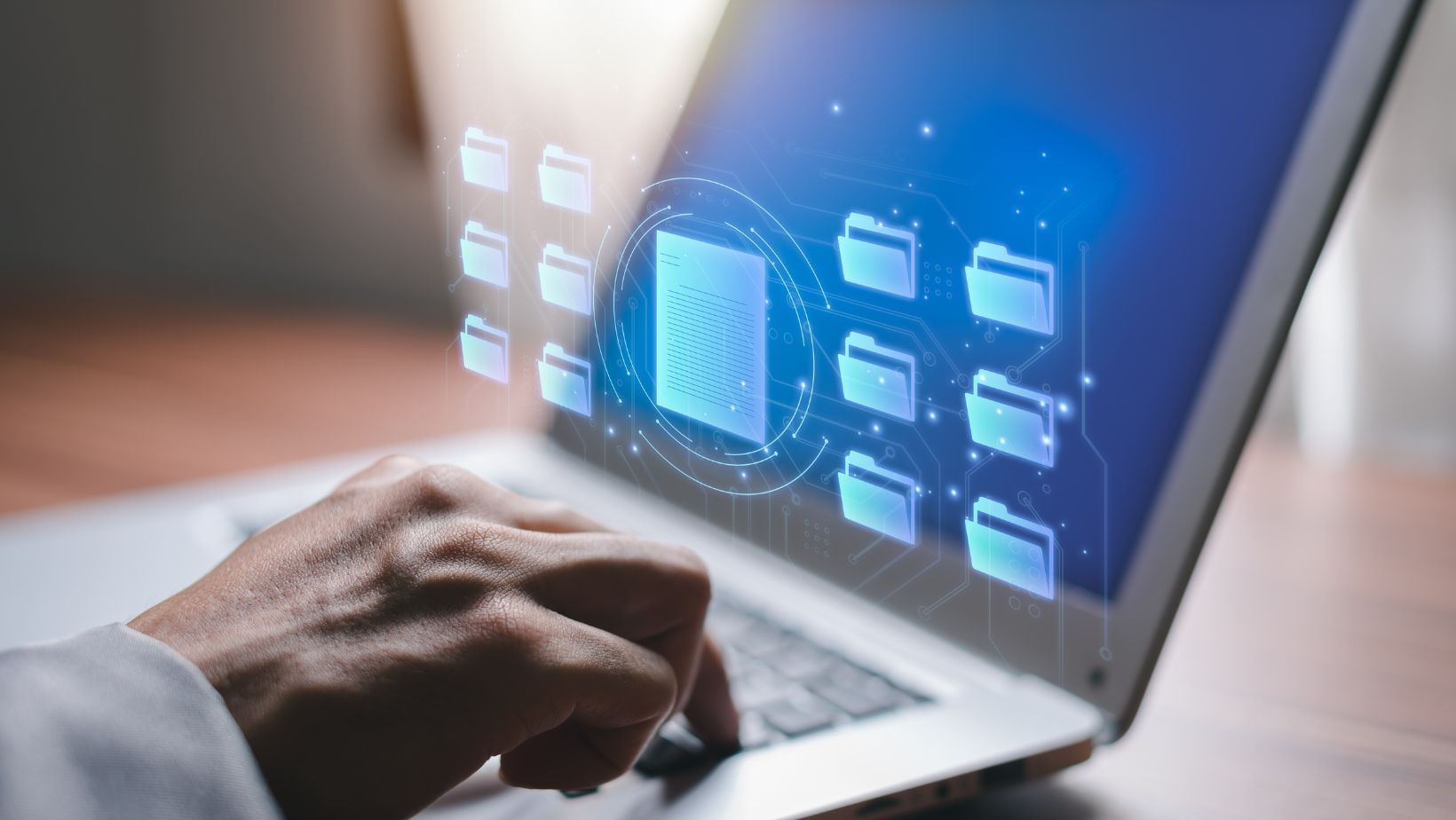
Use Cases and Examples
1. E-commerce Recommendations
Use Case: E-commerce platforms leverage Vector Databases and Vector Search to enhance product recommendations. By analyzing user preferences as vectors, the system can recommend products that align with a customer’s unique tastes, leading to improved user engagement and increased sales.
Example: A customer searching for running shoes might receive recommendations not just based on the keyword “running shoes” but also on the vectors representing their past purchases, browsing history, and preferences, providing more personalized and accurate suggestions.
2. Healthcare Analytics
Use Case: In healthcare, Vector Databases are employed to store and analyze patient data in a high-dimensional space. Vector Search enhances diagnostic accuracy by identifying patterns and similarities within patient records, enabling healthcare professionals to make more informed decisions.
Example: When diagnosing a rare condition, Vector Search can quickly identify similar cases in the database, aiding doctors in devising treatment plans based on successful outcomes in comparable situations.
3. Fraud Detection in Finance
Use Case: Financial institutions use Vector Databases for fraud detection. Transaction data, user behavior, and historical patterns are vectorized, allowing the system to detect anomalies or deviations from established patterns, signaling potential fraudulent activities.
Example: If a credit card transaction deviates significantly from a user’s usual spending patterns, the system can quickly flag it for further investigation, preventing unauthorized transactions in real-time.
4. Content Recommendations in Media Streaming
Use Case: Media streaming services utilize Vector Search to recommend content based on user preferences. By vectorizing user watching habits and content features, the system can offer personalized suggestions, enhancing the overall user experience.
Example: If a user frequently watches science fiction movies and documentaries, the system can recommend similar content, taking into account the vectorized representation of the user’s viewing history.
Conclusion: Paving The Way For Intelligent Data Management
In conclusion, Vector Databases and Vector Search are revolutionizing data management by introducing a more nuanced and efficient approach to information retrieval. Their applications span across industries, from personalized e-commerce experiences to advanced healthcare analytics and fraud detection in finance. As these technologies continue to evolve, businesses are poised to unlock new dimensions of intelligence and innovation in their data-driven endeavors. Embracing the art and science of Vector Databases and Vector Search is not just a technological leap; it’s a strategic move towards a future where data becomes a more accessible, dynamic, and powerful asset.